Sports
Ziye Wang
The analysis in this article was conducted by Formula Bot's Data Analyzer, an AI-powered application that allows users to analyze their data through a simple conversation. No data background necessary.
Get your brackets (and wallets) ready, hoops fans—it’s March Madness time.
As the best college basketball teams in the nation get ready to square off for the sport’s most exciting tournament, many of you might be allowing yourselves to indulge, however briefly, in a fantasy you probably know better than to entertain: “will this year finally be the year I nail my bracket?”
Hate to break it to ya, but the answer’s no, what with the odds of a perfect bracket being somewhere in the ballpark of 1 in 120.2 billion (and that’s if you know your college hoops).
But—with the help of a bit of machine learning trickery, we think there’s a pretty good chance you could at least do better than your friends in your pool. Better yet, we think we might have the secret sauce that could help you nail a Cinderella pick for this year’s tourney; imagine the bragging rights that’ll get you in your group chat.
Curious? Read on to find out how we used a decade’s worth of March Madness results to provide data-driven insights on which lowly seeded teams might just have what it takes to go a little deeper than expected this year (or, vice versa, which powerhouses might crash and burn).
Disclaimer: This analysis is for entertainment and educational purposes only. Remember, no algorithm, no matter how advanced, can outsmart the sheer unpredictability of March Madness. So, while we hope our insights help you gain an edge (or at least some serious bragging rights), please gamble responsibly.
Data collection
As we know, better seeded teams generally have higher odds of making it far in the tournament. Thus, with no other information to go on, picking the better seed is generally the best bet. But this is March Madness we’re talking about, where, on average, 9 upsets happen per tournament. Indeed, picking the most likely suspect for an upset is exactly what we’re trying to do here.
Therefore, to get any predictive insight beyond seeding, we’ll need other metrics, and lots of them.
To collect these metrics, we scraped historical data (i.e., data for seasons 2012 through 2023) from two different sources: sports-reference and kenpom.
KenPom data, created by Ken Pomeroy, is an analytics tool widely used in college basketball for performance evaluation. It considers the efficiency of teams, adjusted for pace and strength of schedule.
From the former, we obtained and computed the following metrics for our model: win-loss %, simple rating system (SRS), strength of schedule (SOS), FG%, 3P%, FT%, home win rate, away win rate, conference win rate and point differential %. From the latter, we obtained the following advanced statistics: adjusted efficiency margin (AdjEM), adjusted offensive efficiency (AdjO), adjusted defensive efficiency (AdjD), adjusted tempo (AdjT), luck, adjusted strength of schedule (SOS AdjEM), average adjusted offensive efficiency of opposing teams (OppO), average adjusted defensive efficiency of opposing teams (OppD) and non-conference adjusted strength of schedule (NCSOS AdjEM). We’ll describe some of these advanced stats in more detail later.
Dataset can be downloaded here for you viewing pleasure.
Model Selection / Approach
After collating the historical data from our two sources into a single training dataset, we used a machine learning model called Random Forest Classification to calculate the likelihood of every team reaching the Sweet Sixteen (i.e., our outcome or target variable) based on 20 metrics in our dataset (i.e., our predictors/metrics).
We then scraped the same data for 2024 (minus, of course, our target variable of reaching the Sweet Sixteen) and ran it through the model we had trained to generate the likelihood for each team making it into the top 16 this year.
What metrics matter?
After training the model on the historical data (2012-2023), we were able to understand, of all the variables, which ones are most influential in terms of resulting in a team reaching the Sweet Sixteen. Some metrics were less irrelevant than others. For example, a team’s free throw shooting percentage is less important than their point differential.
Of all of the metrics, here are the top five most influential metrics, ranked from most important to least:
AdjEM
SRS
Seed
SOS AdjEM
AdjO
Seeding, as we might expect, was one of the most important metrics for making the Sweet Sixteen—but it wasn’t the top one. That distinction went to AdjEM, an advanced metric developed by Ken Pomery that takes the difference between AdjO (points scored per 100 possessions, adjusted for opponent strength) and AdjD (points allowed per 100 possessions, adjusted for opponent strength).
Across over a decade of March Madness, this holistic efficiency measure was a better predictor of a deep tourney run than seeding. Generally speaking, then, if you’re looking to make a blind bet, it might be worth taking that extra bit of effort to look up each team’s AdjEM instead of just going for the better seed.
Predicted probabilities
As supporting data, below is the correlation of every metric to the probability that a team would reach the Sweet Sixteen.
We can see that seeding has the highest negative correlation (the lower the seed, the higher the probability), whereas AdEM, SRS and SOS (Kenpom adjusted stats) have the strongest positive correlation.

Validation
Before applying the model’s weights to the 2024 tournament teams, we were curious to check its accuracy versus last season’s results.
The model made several intriguingly accurate predictions. For instance, it forecasted Purdue, despite being a top seed, with only a 22% chance of reaching the Sweet Sixteen, contrary to the average of 77% for top seeds. Purdue's upset loss to FDU in the first round confirmed this prediction. Conversely, the model foresaw FAU and Princeton making it to the Sweet Sixteen despite their low seeds - 9th and 15th, respectively. Princeton, in particular, defied expectations with a 59% predicted chance, far surpassing the 12% average success rate for its seed. Remarkably, both teams defied the odds and advanced to the Sweet Sixteen stage.
2024 Predictions
Now let’s move on to the fun part: applying our model to this year’s March Madness data.
Below, you’ll find a chart of all 68 teams that qualified for the tournament this season and their respective probability of reaching the Sweet Sixteen - sorted by their seeding.
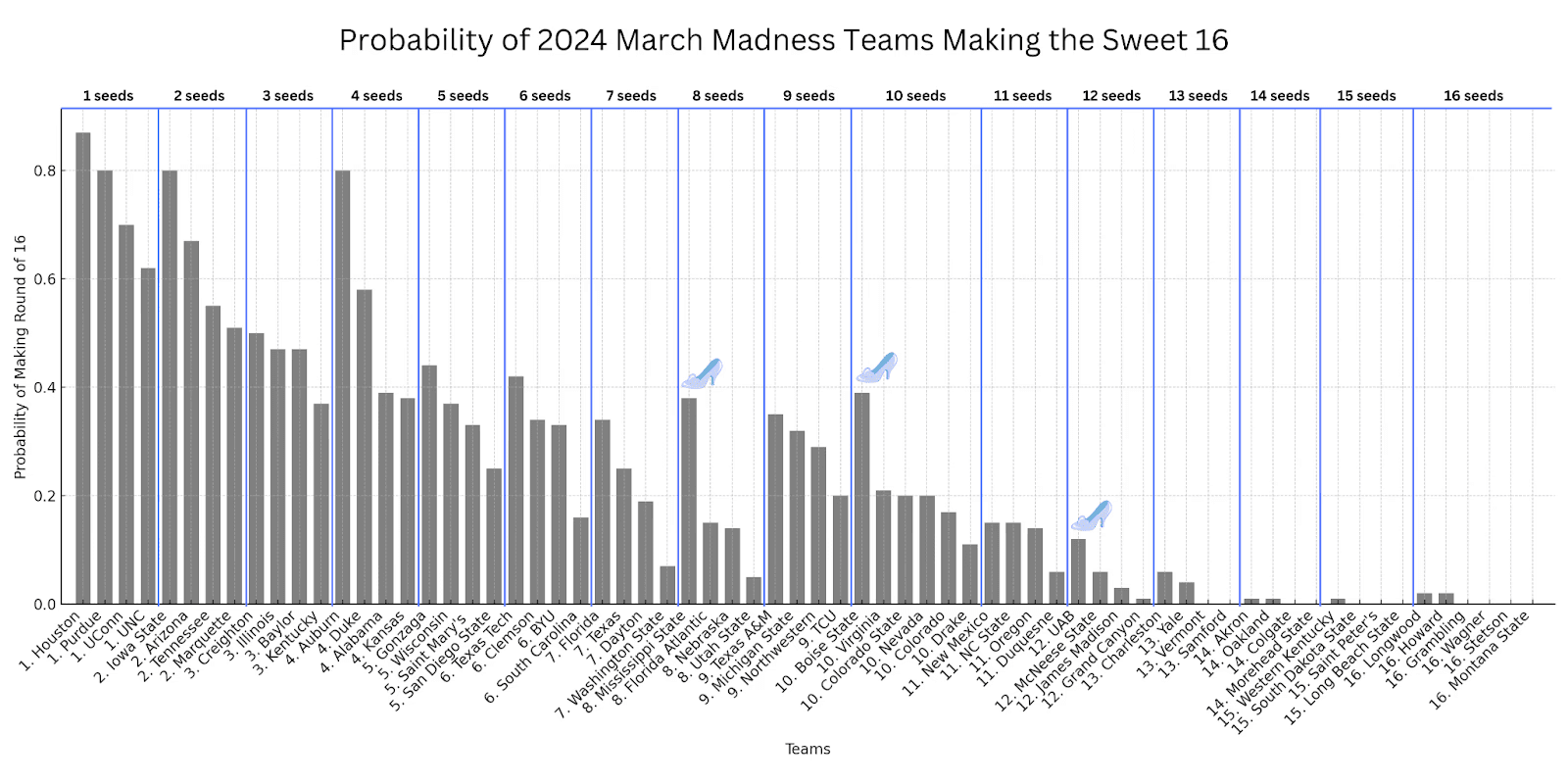
Immediately, you’ll notice that, on average, better seeds tend to have higher probabilities of making the round of 16.
You’ll also find that there’s quite a bit of intra-seed variability due to teams having better or worse efficiency metrics like AdjEM.
This intra-seed variability is how we detect potential underdog stories. We’ve highlighted a few of our top candidates with a Cinderella slipper:
Predicted Cinderella stories:
12th-seeded UAB
10th-seeded Boise State
8th-seeded Mississippi State
These teams boast higher probabilities of reaching the Sweet Sixteen compared to their average respective seedings.
Additionally, though not likely to be regarded as an underdog story, it’s also interesting to note that fourth-seeded Auburn has a probability that more closely resembles a one seed.
Conclusion
To cap things off, let’s reiterate a very important point: we’re dealing with probabilities here. No machine learning model, no matter how advanced, can fully capture the randomness inherent to March Madness.
Still, if you’re in the habit of hastily filling out brackets based on seeding alone while throwing in a couple of arbitrary upsets, the insights provided by our model (specifically, the importance of AdjEM) might just give you a small competitive edge.
With that being said, let the Madness begin!
Digital Marketing
Google Search Console vs Google Analytics
Sports
What's happening beneath the surface of the college football transfer portal
Sports
Do NBA Draft Combine Metrics Predict NBA Success?
Sports
Which college teams are best at preparing players for the NFL? See where your team ranks.
Sports